Cios Grapple With Subpar Global Genai Models
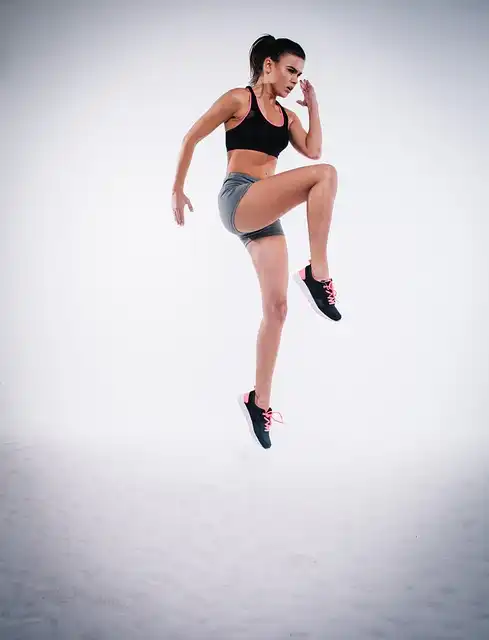
Evan Schuman has covered IT concerns for a lot longer than he’ll ever before admit. The founding editor of retail modern technology website StorefrontBacktalk, he’s been a writer for CBSNews.com, RetailWeek, Computerworld and eWeek and his byline has appeared in titles ranging from BusinessWeek, VentureBeat and Lot Of Money to The New York City Times, USA Today, Reuters, The Philly Inquirer, The Baltimore Sun, The Detroit News and The Atlanta Journal-Constitution. Evan can be gotten to at eschuman@thecontentfirm.com and he can be complied with at http://www.linkedin.com/in/schumanevan/. Try to find his blog site two times a week.
“The volume, splendor, and variability in the underlying training data is vital to getting top quality runtime performance of the version.” Inquiries in languages that are underrepresented in the training data are most likely to yield bad performance, he said.
“As a German citizen who works mostly in English, I’ve discovered that while LLMs are skilled in German, they do not fairly get to native-level proficiency,” stated Vincent Schmalbach, an independent AI engineer in Munich.
Amazon’s Bedrock Market does something comparable, supplying access to a large number of genAI model makers globally. Although it definitely does not reduce every one of the disadvantages of utilizing a little-known supplier in different geographies, Philomin suggests that it addresses a few of them.
How much smaller are the datasets utilized in non-English versions? That differs widely depending on the language. It’s not so much a matter of the number of people that talk that language as it is the volume of data in that language readily available for training.
Using exclusive resources of information– including those in different nations around the globe– will potentially boost the data high quality for some topics and markets, and at the very same time boost the amount of great training data readily available for non-English designs. As the overall cosmos of training information increases, the imbalance in the amount of training data across languages may matter less and less. This shift is additionally most likely to increase prices as the design manufacturers cut deals with third celebrations to certify their private details.
An additional element that might lessen the dataset dimension problem in the next few years is an expected rise in disorganized information. Undoubtedly, extremely unstructured data– such as that collected by video clip drones seeing services and their customers– can potentially avoid language concerns completely, as the video evaluation might be captured straight and saved in several languages.
Allow’s claim a global CIO is getting 118 designs from an LLM supplier, in a large range of languages. The supplier does not tell the CIO just how little training was done on all of those non-English models, and definitely not where that training information came from.
One variable now entering into play lies in the distinction in between public and exclusive information. An executive at one of the biggest design manufacturers– who asked to not be identified by name or employer– claimed the significant LLM makers have pretty much captured as much of the information on the public web as they can. They are remaining to collect new information from the internet daily, of course, however those companies are changing much of their data-gathering efforts to exclusive sources such as colleges and firms.
“Most business are even more thinking about sourcing their structure versions from their relied on companies,” which are typically the major hyperscalers, Curran stated. “Enterprises really intend to acquire those [design training] abilities via their implementations on AWS, Google, or Microsoft. That provides [CIOs] a higher comfort level. They are hesitant to deal with a startup.”.
It starts throughout the procurement process. IT operations people typically ask outstanding inquiries concerning LLMs before they acquire, they have a tendency to be extremely focused on the English variation. It does not occur to them that the quality provided in the non-English models may be dramatically lower.
Given the massive quantity of cash enterprises are spending on genAI, the carrot is apparent. The stick? Maybe CIOs require to leave their convenience area and start getting their non-English models from regional suppliers in every language they require.
Dataset dimension is extremely vital in a genAI version, information high quality is also important. Even though there are no unbiased benchmarks for examining data quality, specialists in different topics have a rough sense of what negative and great material resembles. In medical care, as an example, it could be the distinction in between using the New England Journal of Medication or Lancet versus scuffing the personal website of a chiropractic doctor in Milwaukee.
There’s reason to wish that the disparity in the high quality of result from designs in various languages may be minimized or perhaps negated in the coming years. Because a version based on a smaller dataset may not experience from reduced precision if the underlying data is of a greater high quality, that’s.
One more popular countermeasure for non-English genAI versions is making use of synthetic information to supplement the real data. Artificial data is normally created by machine discovering, which theorizes patterns from actual data to create likely data.
“If you want your language model to be multilingual, the ideal point you can do is have parallel data in the languages you desire to support,” stated Mary Osborne, the senior item manager of AI and natural language processing at SAS. Another preferred countermeasure for non-English genAI designs is making use of synthetic data to supplement the real information. An exec at one of the largest version manufacturers– that asked to not be determined by name or company– stated the significant LLM manufacturers have pretty much recorded as much of the data on the public internet as they can. Touching right into exclusive sources of details– including those in different countries around the globe– will potentially enhance the information high quality for some sectors and topics, and at the exact same time boost the quantity of great training data available for non-English versions. The vendor doesn’t inform the CIO how little training was done on all of those non-English models, and absolutely not where that training data came from.
With the variety of generative AI tests rising in the venture, it is normal for the CIO to buy countless big language models from different version makers, tweaked for various geographies and languages. CIOs are finding that non-English versions are faring much extra badly than English ones, also when purchased from the very same supplier.
“It is almost ensured that all LLM applications in languages apart from English will do with much less precision and less significance than executions in English due to the large variation in training example size,” said Akhil Seth, head of AI service development at expert firm UST.
There is no accurate means to predetermine exactly how much information is offered for training in an offered language, Hans Florian, a distinguished research study scientist for multilingual natural language handling at IBM, has a trick. “You can consider the variety of Wikipedia pages because language. That associates quite well with the amount of data offered in that language,” he stated.
That claimed, fine-tuning can just help a lot. The training data is the heart of the genAI brain. Much more fine-tuning can be similar to attempting to save a salad with rotting spinach by putting on more salad clothing if that is poor.
AWS’s Philomin claimed his group is attempting to divide the difference for IT customers by using a genAI marketplace method, borrowing the technique from the AWS Marketplace– which subsequently had actually obtained the idea from its Amazon parent firm. Amazon’s retail method enables individuals to buy from little merchants through Amazon, with Amazon taking a cut.
“For critical German-language material, I’ve established a sensible process. I connect with the LLM in English to get the finest quality outcome, then equate the outcome to German. This method constantly creates much better results than working directly in German.”.
CIOs can think about sourcing their non-English models from regional/local genAI companies that are indigenous to that language. That method may address the issue for lots of locations, it is going to satisfy solid resistance from numerous enterprise CIOs, said Rowan Curran, an elderly expert for genAI approaches at Forrester.
“However if you wished to include a rare aboriginal language like Cree or Micmac, those languages would be greatly underrepresented in the example. They would yield bad outcomes compared to English and French, due to the fact that the version wouldn’t have actually seen sufficient information in those indigenous languages to do well,” she stated.
Till the volume of top quality information for non-English languages gets much more powerful– something that could slowly happen with more disorganized, private, and language-agnostic information in the following few years– CIOs require to require much better answers from model suppliers on the training information for all non-English versions.
Less information provides less comprehensiveness, much less precision, and a lot more frequent hallucinations. (Hallucinations typically take place when the version has no details to respond to the query, so it makes something up. Proud algorithms these LLMs can be.).
Like dataset dimension, information high quality usually differs by location, according to Jürgen Bross, elderly research study researcher and manager in multilingual at IBM. That implied that, on average, the readily available Japanese data was of reduced high quality.
“If you want your language design to be multilingual, the most effective point you can do is have identical data in the languages you want to support,” stated Mary Osborne, the senior item supervisor of AI and natural language handling at SAS. “That’s a simple proposition in places like Quebec, for example, where all their government data is produced in both English and French. If you intended to have an LLM that did a fantastic job of responding to inquiries about the Canadian federal government in both English and French, you would certainly have a good supply of information to pull that off,” Osbourne claimed.
“There is a raising portion of unstructured content on the internet that is presently developed by generative AI designs. If not mindful, future models can be increasingly educated on outcome from other models, possibly intensifying prejudices and mistakes,” Villanustre stated.
Vasi Philomin, the VP and basic manager for generative AI at Amazon Internet Provider (AWS), among the leading AI as a Solution vendors, estimated that the training datasets for non-English models are roughly “10 to 100 times smaller” than their English equivalents.
Even multilingual designs experience from this,” Seth said.
That caution increases the concern of just how much assistance the AWS reseller role will be if something later strikes up.
And assigning additional spending plan to adjust versions can be tough since the variety of variables– such as the particular languages, subjects, and industry concerned– is too various to provide any kind of reasonable assistance. IBM’s Florian does supply a small bit of positive outlook: “You don’t require a permanent spending plan boost. It’s simply a single spending plan boost, a single expenditure that you take.”.
Jason Andersen, a VP and principal analyst with Moor Insights & Approach, stated CIOs need to do whatever they can to get version makers to share more details about training information for every single version being bought or certified. “There needs to be a lot more openness of data provenance,” he said.
The method that the majority of genAI experts settle on is that CIOs require to budget plan more cash to examination and tweak every non-English version they wish to utilize. That cash additionally needs to cover the additional processing and verification needed for non-English designs.
UST’s Seth said the dataset tests with non-English genAI versions are not mosting likely to be very easy to get rid of. A few of the much more noticeable mechanisms to deal with the smaller sized training datasets for non-English designs– consisting of automated translation and much more aggressive fine-tuning– come with their very own downsides.
The significant version manufacturers– OpenAI, Microsoft, Amazon/AWS, IBM, Google, Anthropic, and Perplexity, among others– do not normally disclose the volume of data each model is educated on, and certainly not the top quality or nature of that information. Enterprises usually take care of this lack of transparency regarding training information through substantial testing, yet that testing is usually concentrated on the English language version, not those in various other languages.
1 data2 models
3 non-English models
4 training data
« 9 Google Chrome features you really should be usingGrubHub got hacked. Go change your password! »