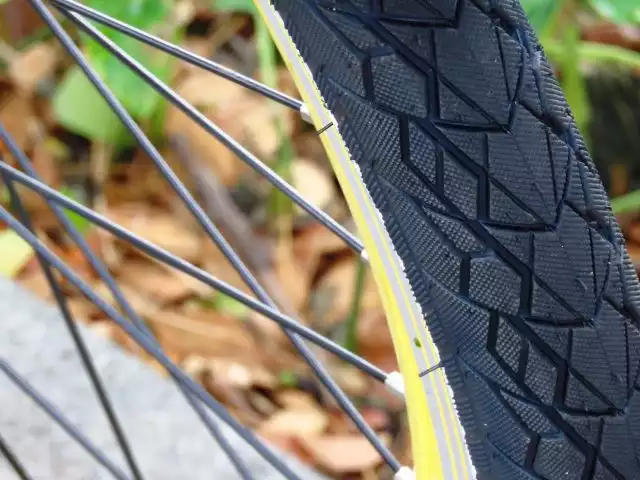
Outcomes for B200 were sent in the “sneak peek” classification, which means Nvidia anticipates Blackwell to be on the market in 6 months or less. Because of both firms’ product tempos, AMD’s present generation MI300X will likely come up against Nvidia’s B200 shortly. For Llama2-70B inference, B200 is around 4 × faster than MI300X today.
Untether’s outcomes actually radiate is power effectiveness. For ResNet-50, 6x accelerators on the slim cards (75 W each) can inference 314 inquiries per 2nd per watt in web server mode, versus 96 for 8x Nvidia H200-141GB– providing Untether around 3 × the power efficiency versus Nvidia’s current generation hardware.
Untether’s 6x “Slim” PCIe cards– which match 2U, each with a solitary accelerator powered at 75 W– can manage 309,752 ResNet-50 inf/sec in server setting, or 334,462 in offline setting. This is around half the performance of an 8x Nvidia H100-SXM-80GB system as submitted by Supermicro, though the Supermicro system is two times as large at 4U and its TDP is more than 10x. Stabilizing per accelerator reveals one Untether speedAI240 has around 65% the efficiency of an H100 in this arrangement. Keep in mind, Untether does not utilize HBM– its accelerators use up to 64 GB LPDDR5 memory with bandwidth 100 GB/s.
Nvidia was also tested by start-up Untether, revealing its very first MLPerf criteria in which its SpeedAI accelerator beat different Nvidia chips on power effectiveness for ResNet-50 work. Google additionally sent outcomes for Trillium, its sixth-generation TPU, and Intel showcased its Granite Rapids CPU for the very first time.
These outcomes are really similar (within 3-4%) of Nvidia’s outcomes for H100-80B on the same work for 8-chip systems. Compared to Nvidia’s H200-141GB, which is properly the H100 with even more and faster memory, AMD is a lot more like 30-40% behind.
AMD has actually placed its 12-chiplet MI300X GPU straight against Nvidia’s H100 and is widely seen as one of the most appealing business offerings to challenge group environment-friendly’s hold on the market. MI300X has more HBM capability and transmission capacity than Nvidia’s H100 and H200 (MI300X has 192 GB with 5.2 TB/s versus H200’s 141 GB at 4.8 TB/s), which ought to be obvious in the results for inference of huge language models (LLMs).
AMD sent its initial outcomes for its Nvidia-challenging information center GPU, the MI300X, revealing its performance in solitary and 8-chip systems for Llama2-70B reasoning. A single MI300X can inference 2520.27 tokens/s in server mode or 3062.72 tokens/s in offline setting, while 8x MI300Xs can do 21,028.20 tokens/s in web server setting and 23,514.80 tokens/s in offline setting. The numbers show relatively direct scalability in between system dimensions. (As a suggestion, the offline circumstance permits batching to take full advantage of throughput, while the more difficult server situation simulates real-time questions with latency limits to satisfy.).
Results for B200 were sent in the “preview” category, which indicates Nvidia expects Blackwell to be on the market in 6 months or less. Nvidia and its partners show off outcomes for the brand-new blend of specialists workload, Mixtral-8x7B.
As soon as again revealed off its CPUs for AI reasoning (no entries from Habana this time), Intel. It showed Granite Rapids, its next-gen Xeon web server CPU with all performance cores (P-cores, versus effectiveness cores or E-cores). Granite Rapids offers 1.9 × the performance of previous generation Xeon CPUs– this is an ordinary throughout all the work sent, that includes just the smaller sized designs, up to and including GPT-J (6B).
Google showed results under “sneak peek” for its next-gen TPUv6e, Trillium, which will launch later this year. Trillium can inference StableDiffusion at 4.49 queries/s in server mode or 5.44 samples/s in offline setting. Versus the current-gen TPUv5e in the exact same round, it roughly triples performance. For comparison, Nvidia GH200 (Grace Receptacle 144 GB) can do 2.02 queries/s in web server setting and 2.30 samples/s in offline mode– concerning fifty percent of Trillium’s efficiency.
Startup Untether flaunted its first MLPerf outcomes, sending performance and power scores for its second-gen speedAI240 accelerator in numerous various system setups. This is a 2-PFLOPS accelerator with more than 1400 RISC-V cores, designed for power efficient AI reasoning.
Nvidia and its partners flaunt results for the new combination of professionals workload, Mixtral-8x7B. This workload has 46.7 B overall criteria with 12.9 energetic per token (mix of specialists models designate queries to among, in this case, eight smaller sized sub-models, called “specialists”). For Mixtral, H200 beat H100 in the very same power envelope (700W); H200 has 1.8 × even more memory with 1.4 × even more data transfer yet provides around 11-12% more efficiency.
Google said Trillium is expected to raise peak calculate efficiency 4.7 × contrasted to its previous generation, as a result of bigger matrix-multiply devices and faster clock rate. HBM capacity and data transfer has actually likewise increased and the bandwidth in between chips has increased as well, as a result of customized optical interconnects.
For the very first time, the current round of MLPerf inference benchmarks includes outcomes for AMD’s flagship MI300X GPU. The opposition revealed comparable results to market leader Nvidia’s H100/H200 existing generation equipment, though Nvidia won in general– yet only by a limited margin.
A solitary B200 in web server mode can inference 10,755.60 tokens/s for Llama2-70B, as much as 4x faster than an H100, or 11,264.40 tokens/s in offline mode, approximately 3.7 x faster than H100. Note that Nvidia has actually quantized to FP4 for the very first time in these results (submitters can quantise as strongly as they like, given they fulfill a stringent precision target, which in this situation was 99.9%). Versus the H200 (with bigger and faster memory than H100, and quantised to FP8), the difference was extra like 2.5 × in both situations. Nvidia did not claim whether it quantized the entire workload to FP4; its transformer engine software application enables combined precision for optimal results during training and reasoning.
Nvidia debuted Blackwell B200 this round. This is the very first GPU with the brand-new Blackwell design; it has twice as much compute as H100/200 because of being two reticle-sized calculate pass away, and it also has more memory versus H200’s 141 GB at 180 GB. It is additionally the initial Nvidia GPU to sustain FP4.
AMD additionally previewed its next-gen Epyc Turin CPUs in mix with MI300X; the renovation was rather low at 4.7% in web server mode or 2.5% in offline mode versus the exact same system with a Genoa CPU, yet it sufficed to press the Turin-based system quicker than DGX-H100 by a small amount. AMD Turin CPUs are not on the marketplace yet.
Software-wise, AMD claimed it made extensive use of its composable kernel (CK) library to create efficiency essential bits for things like prefill interest, FP8 decode paged focus and numerous merged bits. It likewise improved its scheduler for much faster decode organizing and much better prefill batching.
Trillium likewise includes a new generation of SparseCore, which accelerates embedding-heavy work by tactically unloading arbitrary and fine-grained access from TensorCores. Google did not submit results for the DLRM standard for either generation of its TPU in this round to show this off.
MI300X has even more HBM capacity and transmission capacity than Nvidia’s H100 and H200 (MI300X has 192 GB with 5.2 TB/s versus H200’s 141 GB at 4.8 TB/s), which need to be apparent in the results for inference of huge language designs (LLMs). Keep in mind that Nvidia has quantized to FP4 for the initial time in these outcomes (submitters can quantise as aggressively as they such as, given they meet a strict precision target, which in this instance was 99.9%). Nvidia did not state whether it quantized the whole workload to FP4; its transformer engine software application allows blended accuracy for ideal results throughout training and reasoning.
Sally has spent the last 18 years writing about the electronic devices sector from London. She has created for Digital Design, ECN, Electronic Specifier: Design, Components in Electronic devices, and lots of even more news publications.
Untether additionally has systems in the sneak peek category (significance they are not on the market yet) based upon a larger single-chip PCIe card that doubles the power readily available to the accelerator to 150 W. This system additionally improves clock frequency a little. Results enhanced 35% in server mode or 26% in offline mode on a per-accelerator basis for ResNet-50. Two cards with each other offer double the performance, demonstrating direct scalability.
1 AMD2 Nvidia
3 offline mode
4 server mode
5 Super Admin mode
« Phone scammers are using faked AI voices. Here’s how to protect yourselfAre refurbished laptops actually more eco-friendly than new laptops? »